Machine Learning on Big Data
It is no longer a secret that big data is a reason behind the successes of many major technology companies. However, as more and more companies embrace it to store, process and extract value from their huge volume of data, it is becoming a challenge for them to use the collected data in the most efficient way.
That’s where machine learning can help them. Data is a boon for machine learning systems. The more data a system receives, the more it learns to function better for businesses. Hence, using machine learning for big data analytics happens to be a logical step for companies to maximize the potential of big data adoption.
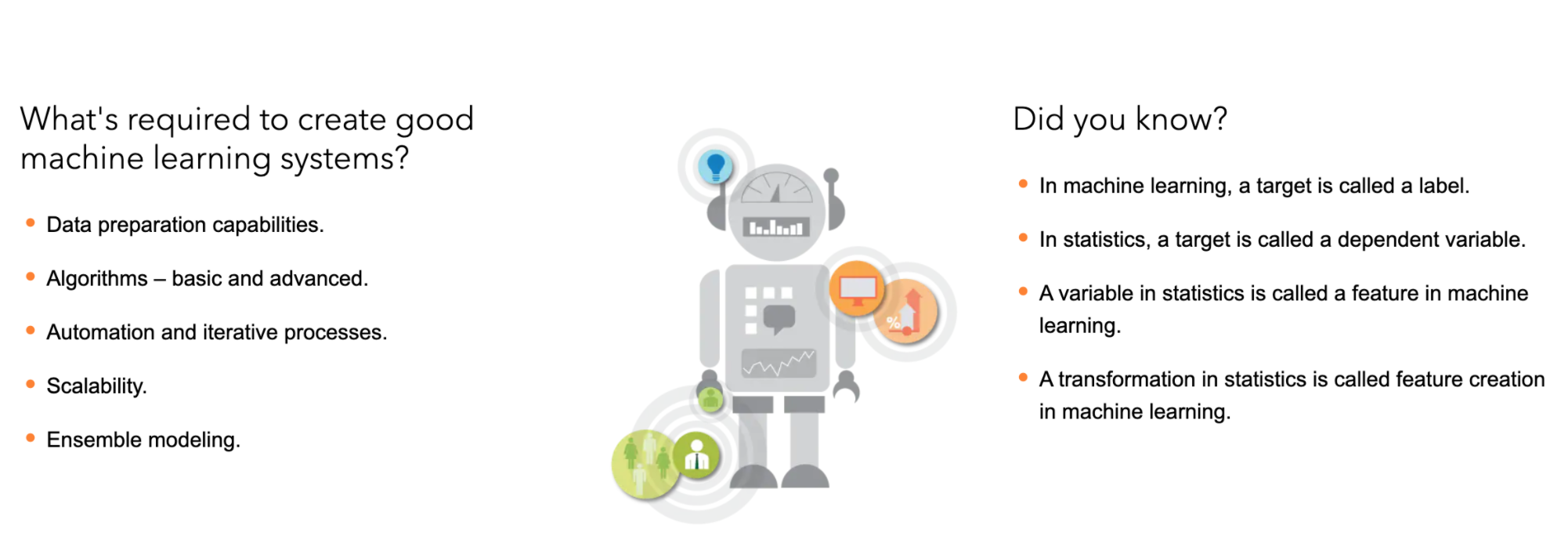
Makes Sense Of Big Data
Big data refers to extremely large sets of structured and unstructured data that cannot be handled with traditional methods. Big data analytics can make sense of the data by uncovering trends and patterns. Machine learning can accelerate this process with the help of decision-making algorithms. It can categorize the incoming data, recognize patterns and translate the data into insights helpful for business operations.
Compatible With All Elements Of Big Data
Machine learning algorithms are useful for collecting, analyzing and integrating data for large organizations. They can be implemented in all elements of big data operation, including data labeling and segmentation, data analytics and scenario simulation.
Carrying out market research and segmentation
The target audience is the cornerstone of any business. Every enterprise needs to understand the audience and market that it wants to target in order to be successful. That is the reason enterprises need to carry out market research that can delve deep into the minds of potential customers and provide insightful data. Machine learning can help in this regard by using supervised and unsupervised algorithms to interpret consumer patterns and behaviors accurately. Media and the entertainment industry use machine learning to understand the likes and dislikes of their audiences and target the right content to them.
Exploring customer behavior
Machine learning does not stop after drawing a picture of your target audience. It also helps businesses explore audience behavior and create a solid framework of their customers. This system of machine learning, known as user modeling, is a direct outcome of human-computer interaction. It mines data to capture the mind of the user and enable business enterprises to make intelligent decisions. Facebook, Twitter, Google and others rely on user modeling system to know their users inside out and make relevant suggestions.
Why is machine learning important?
Resurging interest in machine learning is due to the same factors that have made data mining and Bayesian analysis more popular than ever. Things like growing volumes and varieties of available data, computational processing that is cheaper and more powerful, and affordable data storage.
All of these things mean it’s possible to quickly and automatically produce models that can analyse bigger, more complex data and deliver faster, more accurate results – even on a very large scale. And by building precise models, an organization has a better chance of identifying profitable opportunities – or avoiding unknown risks.
IT Researches can help government organizations:
Detect and prevent fraudulent transactions, accounts, and vendors
Constant improvement of fraud detection capabilities is crucial to addressing rapidly shifting fraud scenarios. Compared to legacy solutions, IT Researches can incorporate and analyse more data across multiple channels, such as point-of-sales, web, and mobile to yield faster, real-time fraud analytics and forensics.
Identify anomalies or signatures to address proliferation, terrorism, money laundering, counterfeit devices, threats, and other criminal activity
Leverage your data assets to make informed decisions and detect suspicious activity before it’s too late.
Identify important information, trends, and triggers to highlight the most relevant and high impact information
IT Researches provides customized views of complex data, refocusing attention onto areas of relevance in relation to the problem at hand, as well as the opportunity to incorporate user feedback without setting hard rules that create permanent knowledge holes moving forward.
Cybersecurity
Identify abnormal activity, correlated nefarious patterns across multiple data types and inputs, and prescribe actions based on all the factors. Examples: insider threat, network design/operations, threat detection/alerting, and software defined networking.
Situational Profiling
Based on the information at hand, identify what is important and where to look. Customized view of complex data. Examples: risk heat map, identity intelligence, activity based intelligence (ABI)/object based production (OBP), and event/activity prediction.
Pattern of Life
Identify trends and correlations among different groups to detect various subtle and complex patterns. Deep customization of response based on thorough understanding of the players involved.
Additional use cases:
Prescriptive Maintenance, Operations, Disease Control & Prevention